High-Performance Machine Learning Automation Software Platform
In today's fast-paced digital landscape, the demand for efficient and scalable machine learning solutions is at an all-time high. High-performance machine learning automation software platforms are revolutionizing how businesses harness data, enabling rapid deployment and seamless integration of AI-driven insights. These platforms empower organizations to streamline workflows, enhance decision-making, and unlock unprecedented levels of innovation, setting a new standard for operational excellence in the era of intelligent automation.
Introduction: The Need for Automated Machine Learning
In today's data-driven world, the demand for machine learning solutions is rapidly increasing. However, developing and deploying machine learning models can be a complex and time-consuming process, often requiring specialized expertise. Automated machine learning (AutoML) platforms are designed to address these challenges by streamlining the workflow and making machine learning accessible to a broader audience. These platforms enable organizations to harness the power of machine learning without the need for extensive technical knowledge.
- AutoML platforms reduce the time and effort needed to build and deploy models.
- They democratize access to machine learning by simplifying complex processes.
- These platforms enable rapid experimentation and iteration, accelerating innovation.
As the volume and complexity of data continue to grow, the need for efficient and scalable machine learning solutions becomes more critical. AutoML platforms empower businesses to leverage machine learning capabilities effectively, improving decision-making and driving competitive advantage. By automating key aspects of the machine learning pipeline, these platforms allow data scientists and business professionals alike to focus on deriving insights and creating value from their data.
Key Features of a High-Performance ML Automation Platform
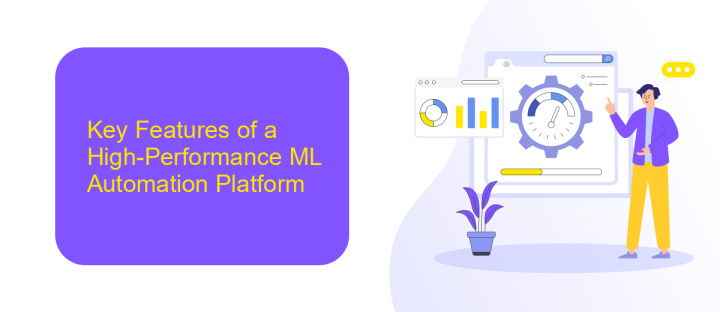
A high-performance ML automation platform is distinguished by its ability to streamline the entire machine learning lifecycle, from data preparation to model deployment. One of its key features is an intuitive user interface that allows users to easily navigate through complex processes without requiring extensive technical expertise. The platform should support a wide range of data sources and formats, enabling seamless integration with existing systems. Advanced automation capabilities, such as hyperparameter tuning and automated feature engineering, significantly reduce the time and effort needed to develop and optimize models.
Another crucial feature is scalability, ensuring that the platform can handle large datasets and complex computations efficiently. Robust security measures must be in place to protect sensitive data and ensure compliance with industry standards. Additionally, the platform should offer integration capabilities with tools like ApiX-Drive, facilitating smooth data transfer and process automation across various applications. Comprehensive monitoring and reporting tools provide insights into model performance and operational metrics, allowing users to make informed decisions and continuously improve their ML workflows.
Architecture and Design Principles for ML Automation
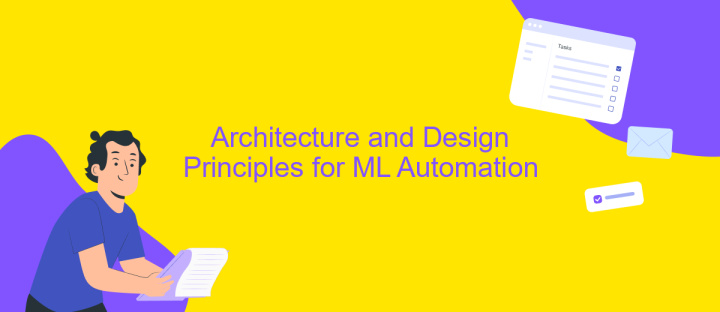
The architecture of a high-performance machine learning automation platform is fundamentally built on scalability, flexibility, and efficiency. It is designed to seamlessly integrate with existing data infrastructure and support diverse machine learning workflows, from data preprocessing to model deployment. The platform should be modular, allowing for easy updates and customization to meet evolving business needs. A user-friendly interface is crucial for facilitating collaboration between data scientists and business stakeholders, ensuring that the platform is accessible to users with varying levels of technical expertise.
- Scalability: The platform should handle large volumes of data and support distributed computing to enhance processing power.
- Flexibility: It must accommodate various machine learning frameworks and algorithms, offering a wide range of tools for different tasks.
- Efficiency: Automation of repetitive tasks, such as data cleaning and model tuning, should be prioritized to accelerate the ML pipeline.
By adhering to these design principles, the platform can effectively streamline machine learning processes, reducing time-to-market for predictive models. This architecture not only supports robust model development but also ensures that the platform remains adaptable to future technological advancements and organizational changes, maximizing long-term value.
Benefits and Use Cases of Automated Machine Learning
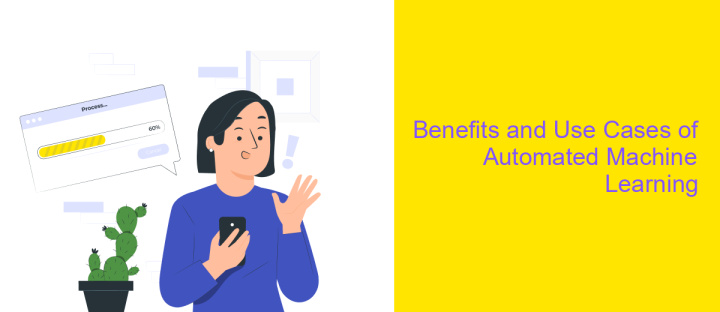
Automated Machine Learning (AutoML) has revolutionized the way businesses approach data-driven decision-making. By simplifying the complex processes involved in model selection, training, and optimization, AutoML platforms enable organizations to harness the power of machine learning without requiring extensive expertise in the field.
One of the primary benefits of AutoML is its ability to streamline workflows and reduce the time required to deploy effective models. This efficiency allows data scientists and analysts to focus on higher-level strategic tasks, enhancing productivity and innovation within teams.
- Rapid prototyping and deployment of machine learning models.
- Increased accessibility for non-experts, democratizing data science.
- Improved model accuracy through automated hyperparameter tuning.
- Scalability across various industries, from finance to healthcare.
In real-world scenarios, AutoML proves invaluable in areas such as predictive maintenance, where it can forecast equipment failures, and in customer segmentation, where it enables personalized marketing strategies. As AutoML technology continues to evolve, its applications will expand, offering even more opportunities for businesses to leverage machine learning effectively.
- Automate the work of an online store or landing
- Empower through integration
- Don't spend money on programmers and integrators
- Save time by automating routine tasks
Choosing the Right ML Automation Platform: Evaluation Criteria
When selecting a machine learning automation platform, it's crucial to evaluate its scalability and flexibility. A robust platform should accommodate growing data volumes and support diverse machine learning models and workflows. Assess whether the platform offers a user-friendly interface and comprehensive documentation to facilitate ease of use for both beginners and experienced data scientists. Additionally, consider the platform's capability to integrate with existing tools and systems. Seamless integration ensures efficient data flow and maximizes productivity. Services like ApiX-Drive can simplify these integrations, allowing you to connect various applications without extensive coding.
Another critical criterion is the platform's support for collaboration and version control. Look for features that enable team collaboration, such as shared workspaces and version tracking, to streamline project management and ensure consistency. Evaluate the platform's security measures to protect sensitive data and ensure compliance with industry standards. Lastly, consider the cost-effectiveness of the platform, weighing the pricing model against the features offered. By thoroughly assessing these criteria, you can choose a machine learning automation platform that aligns with your organizational needs and enhances your data-driven initiatives.
FAQ
What is a High-Performance Machine Learning Automation Software Platform?
How can such a platform benefit my business?
What features should I look for in a High-Performance Machine Learning Automation Software Platform?
How do I integrate such a platform with my existing systems?
What are the security considerations when using a machine learning automation platform?
Routine tasks take a lot of time from employees? Do they burn out, do not have enough working day for the main duties and important things? Do you understand that the only way out of this situation in modern realities is automation? Try Apix-Drive for free and make sure that the online connector in 5 minutes of setting up integration will remove a significant part of the routine from your life and free up time for you and your employees.