What is Azure Data Factory Vs Databricks
In the rapidly evolving world of data engineering and analytics, Azure Data Factory and Databricks are two powerful tools that play pivotal roles. While both are integral to data processing and transformation, they serve distinct purposes and offer unique features. This article delves into the key differences and use cases of Azure Data Factory and Databricks, helping you choose the right tool for your needs.
Introduction to Azure Data Factory and Databricks
Azure Data Factory and Databricks are two powerful cloud-based tools offered by Microsoft Azure, designed to handle large-scale data processing and analytics. Azure Data Factory (ADF) is primarily focused on data integration and orchestration, enabling users to create, schedule, and manage data pipelines. On the other hand, Databricks is a unified analytics platform, built on Apache Spark, that offers advanced analytics and machine learning capabilities.
- Azure Data Factory: Facilitates data movement and transformation across various data stores.
- Databricks: Provides a collaborative environment for data scientists, engineers, and analysts to perform data analysis and machine learning.
Both services are essential for modern data-driven enterprises, providing complementary functionalities. While Azure Data Factory excels in orchestrating data workflows, Databricks shines in data processing and analytics. For seamless integration, services like ApiX-Drive can be utilized to automate data transfers and streamline workflows between ADF, Databricks, and other applications, enhancing overall efficiency and productivity.
Use Cases for Azure Data Factory and Databricks
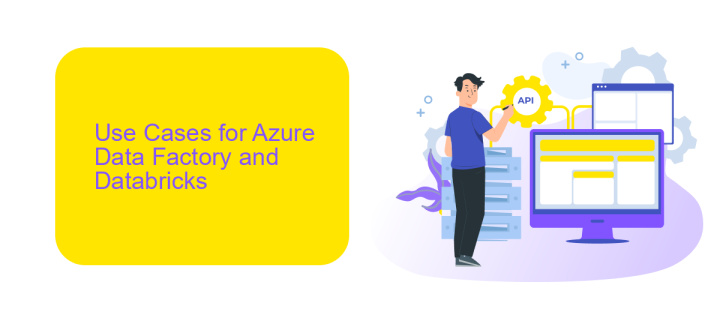
Azure Data Factory (ADF) is ideal for orchestrating and automating data workflows. It excels in scenarios requiring data extraction, transformation, and loading (ETL) from various sources to destinations. ADF is particularly useful for integrating on-premises data with cloud data services, enabling seamless data migration and synchronization. For instance, businesses can use ADF to move and transform large datasets from SQL databases to Azure Data Lake Storage, ensuring data is consistently updated and accessible for analysis.
Databricks, on the other hand, is designed for big data processing and advanced analytics. It is highly effective in scenarios involving large-scale data processing, machine learning, and real-time analytics. Databricks integrates seamlessly with Apache Spark, making it suitable for data engineering and data science workflows. For example, companies can leverage Databricks to perform complex data transformations, build machine learning models, and analyze streaming data in real-time. Additionally, services like ApiX-Drive can be used alongside Databricks to automate data integration from various APIs, further enhancing data processing capabilities.
Comparison of Azure Data Factory and Databricks
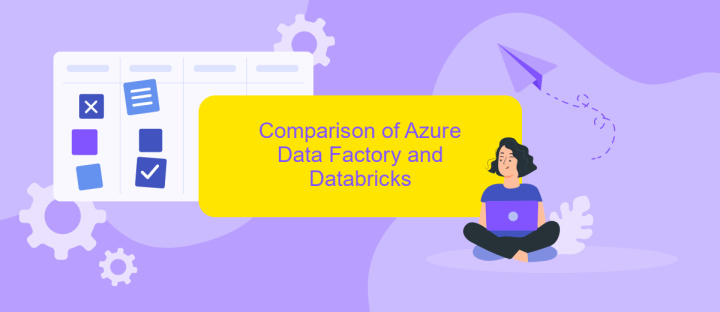
Azure Data Factory and Databricks are both powerful tools for data integration and processing, each with its own strengths. Azure Data Factory is a cloud-based data integration service that allows you to create data-driven workflows for orchestrating and automating data movement and data transformation. Databricks, on the other hand, is a unified analytics platform that provides an interactive workspace for collaboration among data scientists, data engineers, and business analysts.
- Data Integration: Azure Data Factory excels in data integration with its wide range of connectors and seamless integration capabilities.
- Data Processing: Databricks offers robust data processing capabilities with its support for Apache Spark, enabling large-scale data analytics and machine learning.
- User Interface: Azure Data Factory provides a user-friendly visual interface for designing workflows, while Databricks offers an interactive notebook environment.
- Collaboration: Databricks is designed for collaborative work, making it easier for teams to work together on data projects.
- Integration Services: Tools like ApiX-Drive can be used to enhance integration capabilities, providing additional flexibility and automation options for both platforms.
In summary, Azure Data Factory is ideal for orchestrating and automating data workflows, particularly for ETL processes, while Databricks is better suited for advanced data analytics and collaborative data science projects. Depending on your specific needs, you may choose one or even use both in conjunction to leverage their unique strengths.
Choosing Between Azure Data Factory and Databricks
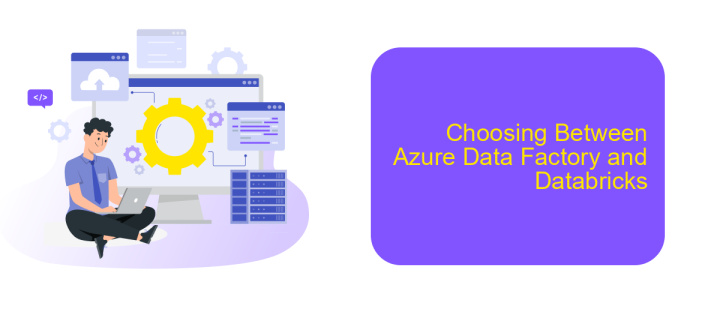
Choosing between Azure Data Factory (ADF) and Databricks depends on your specific data processing needs. ADF excels in orchestrating and automating data workflows, making it a preferred choice for ETL (Extract, Transform, Load) processes. It provides a user-friendly interface and seamless integration with various data sources.
On the other hand, Databricks is designed for big data analytics and machine learning. It offers a collaborative environment for data scientists and engineers to work on large datasets using Apache Spark. Databricks is ideal for advanced analytics and real-time data processing tasks.
- Use Azure Data Factory for orchestrating and automating ETL workflows.
- Choose Databricks for big data analytics and machine learning tasks.
- Consider ADF for seamless integration with various data sources.
- Opt for Databricks when working with large datasets and real-time data processing.
Additionally, tools like ApiX-Drive can enhance your data integration capabilities by simplifying the connection between different services and automating data transfer. This can be particularly useful when working with either ADF or Databricks, ensuring smooth and efficient data workflows.
Conclusion
In conclusion, both Azure Data Factory and Databricks offer robust solutions for data integration and processing, each with its unique strengths. Azure Data Factory excels in orchestrating data workflows and integrating various data sources, making it a powerful tool for ETL processes. On the other hand, Databricks provides a collaborative environment with powerful analytics and machine learning capabilities, ideal for data engineering and data science tasks.
Choosing between the two depends on your specific needs and existing infrastructure. For seamless integration and automation of data workflows, Azure Data Factory is a great choice. However, if you require advanced analytics and a collaborative workspace, Databricks stands out. Additionally, services like ApiX-Drive can further enhance your integration capabilities by automating data transfers and syncing between different platforms, ensuring a smooth and efficient data pipeline. Ultimately, leveraging the strengths of both platforms can provide a comprehensive solution for modern data management challenges.
- Automate the work of an online store or landing
- Empower through integration
- Don't spend money on programmers and integrators
- Save time by automating routine tasks
FAQ
What is the primary difference between Azure Data Factory and Databricks?
Can Azure Data Factory and Databricks be used together?
Which tool is better for ETL processes, Azure Data Factory or Databricks?
How do I automate data integration workflows between different systems?
Is Databricks suitable for real-time data processing?
Do you want to achieve your goals in business, career and life faster and better? Do it with ApiX-Drive – a tool that will remove a significant part of the routine from workflows and free up additional time to achieve your goals. Test the capabilities of Apix-Drive for free – see for yourself the effectiveness of the tool.