Computational Principles and Challenges in Single Cell Data Integration
Single-cell data integration is a rapidly evolving field that aims to combine diverse datasets to gain comprehensive insights into cellular heterogeneity. This article explores the computational principles underpinning this integration, addressing key challenges such as data alignment, normalization, and scalability. By understanding these principles, researchers can better harness the power of single-cell technologies to unravel complex biological systems.
Introduction
Single-cell technologies have revolutionized our understanding of cellular diversity and function, offering unprecedented resolution to study the complexities of biological systems. However, integrating data from various single-cell experiments presents significant computational challenges. This integration is crucial for comprehensive insights but requires sophisticated methods to address issues such as batch effects, scalability, and heterogeneity.
- Batch effects: Variability introduced by different experimental conditions.
- Scalability: Handling the vast amount of data generated by single-cell experiments.
- Heterogeneity: Accounting for the diverse cell types and states within a dataset.
Addressing these challenges necessitates the development of novel computational frameworks and algorithms. Researchers must leverage machine learning, statistical methods, and high-performance computing to create robust solutions. By overcoming these hurdles, the scientific community can unlock the full potential of single-cell data, paving the way for breakthroughs in personalized medicine, developmental biology, and beyond.
Computational Principles for Single Cell Data Integration
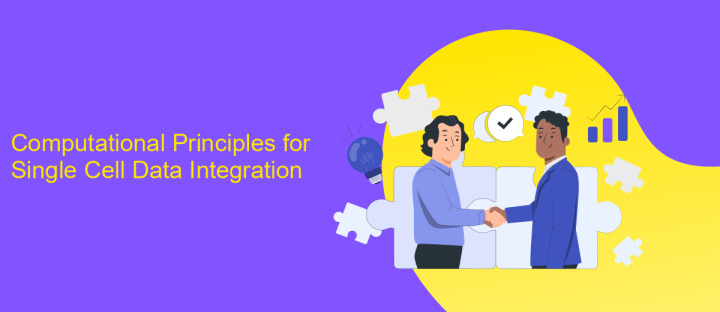
Single cell data integration requires robust computational principles to accurately unify diverse datasets. One key principle is data normalization, which ensures that variations between datasets do not obscure biological signals. Techniques such as batch effect correction and scaling are crucial for aligning data from different sources. Another principle is feature selection, where relevant biological features are identified and used to reduce dimensionality, thus improving computational efficiency and interpretability.
Advanced algorithms like canonical correlation analysis (CCA) and mutual nearest neighbors (MNN) are employed to integrate data by finding common structures across datasets. These methods can be complemented by services like ApiX-Drive, which streamline the integration process by automating data synchronization and transformation. Additionally, visualization tools play a pivotal role in interpreting integrated data, enabling researchers to uncover new biological insights. By adhering to these computational principles, the integration of single cell data becomes more accurate and insightful, facilitating advancements in biological research and personalized medicine.
Challenges in Single Cell Data Integration
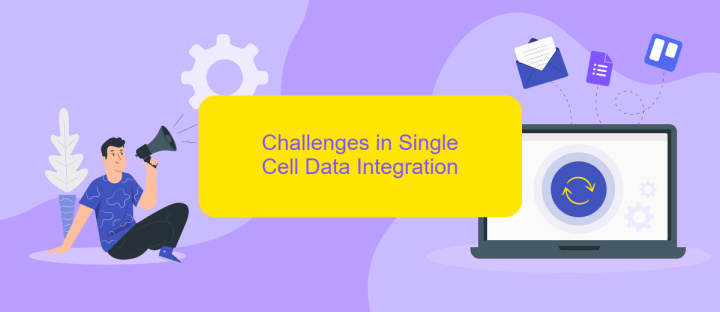
Single cell data integration presents numerous challenges due to the complexity and heterogeneity of biological systems. The need to harmonize data from various sources, each with its own technical biases and noise, adds an additional layer of difficulty. Ensuring the accuracy and consistency of integrated data is paramount for reliable downstream analysis and interpretation.
- Data Heterogeneity: Different single-cell technologies produce data with varying resolutions and scales, complicating integration efforts.
- Batch Effects: Variations introduced during sample processing and sequencing can obscure true biological signals.
- Scalability: Integrating datasets with millions of cells requires substantial computational resources and efficient algorithms.
- Annotation Consistency: Ensuring uniform cell-type annotations across diverse datasets is challenging but crucial for meaningful integration.
- Dimensionality Reduction: Reducing high-dimensional single-cell data while preserving critical information is a significant hurdle.
Addressing these challenges requires the development of robust computational methods and frameworks that can handle the inherent variability and scale of single-cell data. Continuous advancements in computational biology and bioinformatics are essential to overcome these obstacles and fully leverage the potential of single-cell technologies.
Current and Future Directions
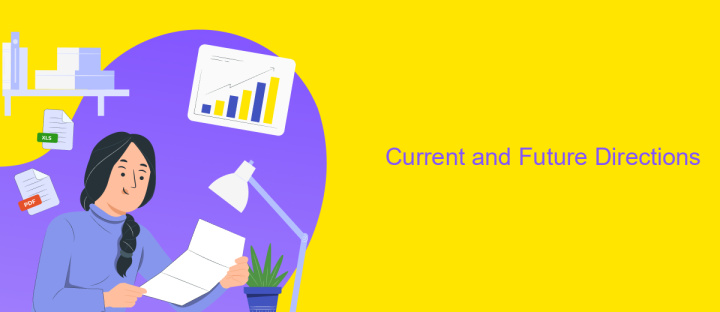
Current advancements in single-cell data integration have significantly improved our understanding of cellular heterogeneity. However, numerous challenges remain, particularly in the realms of data harmonization, scalability, and interpretability. Addressing these issues is crucial for the continued progress in this field.
Future research directions should focus on developing robust computational methods that can handle the increasing complexity and volume of single-cell data. Integrative approaches that combine multi-omics data will be particularly valuable in providing a more comprehensive view of cellular states and dynamics.
- Developing scalable algorithms for large datasets
- Improving methods for data harmonization and normalization
- Enhancing interpretability through advanced visualization tools
- Integrating multi-omics data for holistic cellular insights
By addressing these key areas, the field can move towards more accurate and comprehensive models of cellular function. This will not only enhance our fundamental understanding of biology but also pave the way for novel therapeutic strategies and personalized medicine approaches.
Conclusion
The integration of single-cell data presents both significant opportunities and formidable challenges. Computational principles such as data normalization, feature selection, and dimensionality reduction are crucial for effective integration. These principles help in harmonizing data from diverse sources, enabling comprehensive insights into cellular heterogeneity and function. Despite these advances, challenges such as batch effects, data sparsity, and scalability remain persistent issues that need to be addressed through continuous methodological innovations.
Leveraging integration services like ApiX-Drive can streamline the process of combining disparate datasets. ApiX-Drive offers automated workflows and real-time synchronization, which can significantly reduce the manual effort required for data integration. By utilizing such tools, researchers can focus more on the analytical aspects rather than the technical hurdles, thereby accelerating discoveries in single-cell biology. Future work should aim to refine these computational techniques and explore novel algorithms to further enhance the reliability and efficiency of single-cell data integration.
FAQ
What is single cell data integration and why is it important?
What are the main computational challenges in single cell data integration?
How can batch effects be corrected in single cell data integration?
What tools or frameworks are commonly used for single cell data integration?
Can automation be used to streamline single cell data integration processes?
Apix-Drive will help optimize business processes, save you from a lot of routine tasks and unnecessary costs for automation, attracting additional specialists. Try setting up a free test connection with ApiX-Drive and see for yourself. Now you have to think about where to invest the freed time and money!