ETL vs Big Data
In the evolving landscape of data management, understanding the differences between ETL (Extract, Transform, Load) and Big Data is crucial for businesses aiming to leverage their data assets effectively. While ETL focuses on structured data processing, Big Data encompasses a wider scope, dealing with vast volumes of unstructured data. This article explores the key distinctions and applications of both approaches.
Introduction
In today's data-driven world, businesses need efficient ways to handle and process vast amounts of information. Two prominent methods for managing data are ETL (Extract, Transform, Load) and Big Data technologies. Understanding the differences and applications of these approaches is crucial for making informed decisions about data management strategies.
- ETL: A process that involves extracting data from various sources, transforming it into a suitable format, and loading it into a data warehouse or database.
- Big Data: Technologies and practices designed to handle large volumes of data, often in real-time, to derive insights and support decision-making.
While ETL focuses on structured data and predefined workflows, Big Data encompasses a broader range of data types and processing methods. Tools like ApiX-Drive can facilitate integration and automation, streamlining the data management process. By leveraging the strengths of both ETL and Big Data, businesses can optimize their data workflows and gain a competitive edge.
ETL vs Big Data: Overview and Key Differences
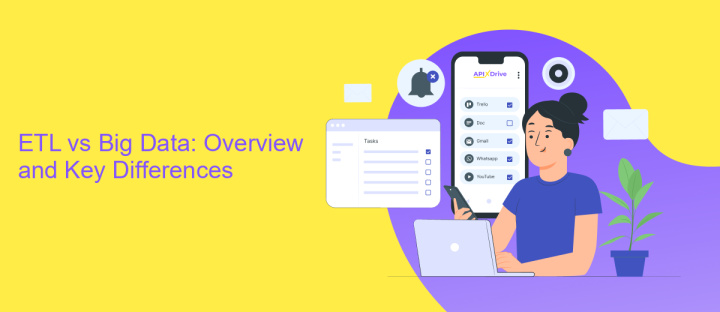
ETL (Extract, Transform, Load) and Big Data are two critical components in the data management landscape, each serving distinct purposes. ETL is a process used to extract data from various sources, transform it into a suitable format, and load it into a data warehouse or other storage systems. This process ensures data is clean, organized, and ready for analysis. On the other hand, Big Data refers to extremely large data sets that require advanced methods and technologies for processing and analysis. Big Data emphasizes volume, velocity, and variety, making it essential for handling complex and large-scale data operations.
One key difference between ETL and Big Data is their focus and application. ETL is primarily concerned with the preparation and integration of data for structured analysis, often in a controlled environment. Big Data, however, deals with unstructured and semi-structured data, utilizing distributed computing frameworks like Hadoop and Spark to process vast amounts of information in real-time. Tools like ApiX-Drive can facilitate ETL processes by automating data integration from multiple sources, streamlining the workflow, and ensuring data consistency. In contrast, Big Data technologies are designed to handle the scale and complexity of data, enabling businesses to derive insights from diverse and rapidly changing data streams.
Comparison of ETL and Big Data Processing
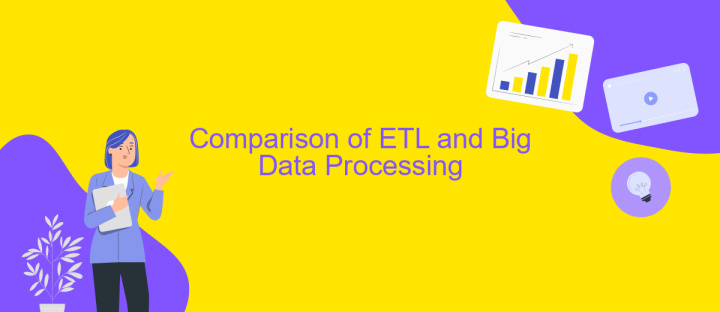
ETL (Extract, Transform, Load) and Big Data Processing are two distinct approaches to managing and analyzing data. ETL is traditionally used for structured data integration and transformation, while Big Data Processing handles vast volumes of unstructured data.
- Data Volume: ETL processes smaller, structured datasets, whereas Big Data Processing manages large-scale, unstructured data.
- Complexity: ETL involves predefined steps, making it less complex. Big Data Processing requires advanced techniques like distributed computing.
- Real-Time Processing: ETL often works in batch mode, whereas Big Data Processing supports real-time analytics.
- Tools: ETL uses tools like Apache Nifi, while Big Data Processing employs frameworks like Hadoop and Spark.
- Integration: Services like ApiX-Drive can simplify ETL integration, enhancing efficiency and connectivity.
While ETL is ideal for traditional data warehousing, Big Data Processing is essential for handling contemporary data challenges. Businesses often leverage both methods to optimize their data strategies, ensuring they can handle both structured and unstructured data efficiently.
Advantages and Disadvantages of ETL and Big Data
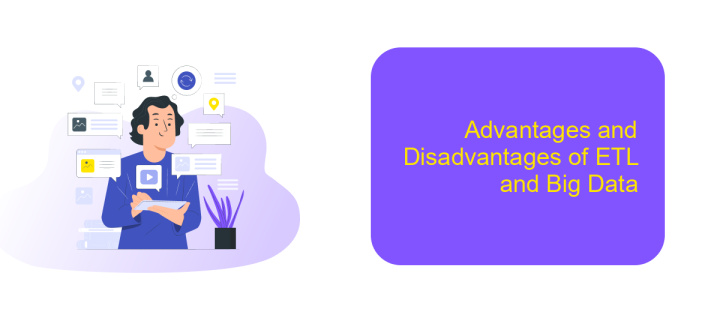
ETL (Extract, Transform, Load) is a traditional data processing method that is highly effective for structured data and well-defined workflows. It allows for precise control over data transformation and integration, making it ideal for enterprise environments with specific data requirements. However, ETL processes can be time-consuming and resource-intensive, often requiring specialized skills and tools.
On the other hand, Big Data technologies excel in handling vast amounts of unstructured data from diverse sources in real-time. They offer scalability and flexibility, enabling businesses to derive insights from complex datasets. Despite these advantages, Big Data solutions can be complex to implement and manage, often requiring significant investment in infrastructure and expertise.
- ETL Advantages: Precise control, structured data handling, ideal for specific workflows.
- ETL Disadvantages: Time-consuming, resource-intensive, requires specialized skills.
- Big Data Advantages: Handles unstructured data, scalable, real-time processing.
- Big Data Disadvantages: Complex implementation, high infrastructure cost, requires expertise.
For businesses looking to streamline their data integration processes, services like ApiX-Drive can be invaluable. ApiX-Drive offers a user-friendly platform for automating data transfers between various applications, reducing the need for manual intervention and allowing for more efficient data management.
Conclusion
In conclusion, both ETL and Big Data play crucial roles in the data management landscape. ETL processes are essential for extracting, transforming, and loading data into a structured format, making it easier for businesses to analyze and derive insights. On the other hand, Big Data technologies enable the handling of vast volumes of unstructured data, providing the scalability and flexibility needed to manage complex data sets.
Choosing between ETL and Big Data depends on the specific needs and goals of an organization. For seamless integration of various data sources, services like ApiX-Drive offer valuable solutions, automating data workflows and ensuring efficient data management. Ultimately, a strategic approach that leverages the strengths of both ETL and Big Data can empower businesses to harness the full potential of their data.
FAQ
What is the main difference between ETL and Big Data?
Can ETL handle Big Data?
What are some common use cases for ETL in Big Data environments?
How can automation improve ETL processes in Big Data?
What are the challenges of integrating ETL with Big Data platforms?
Time is the most valuable resource for business today. Almost half of it is wasted on routine tasks. Your employees are constantly forced to perform monotonous tasks that are difficult to classify as important and specialized. You can leave everything as it is by hiring additional employees, or you can automate most of the business processes using the ApiX-Drive online connector to get rid of unnecessary time and money expenses once and for all. The choice is yours!