Reference Data Integration
Reference data integration is a critical process in modern data management, enabling organizations to unify disparate data sources into a cohesive framework. By harmonizing reference data, businesses can ensure consistency, accuracy, and reliability across various systems and applications. This integration not only enhances data quality but also facilitates better decision-making and operational efficiency, ultimately driving strategic growth and innovation in an increasingly data-driven world.
Understanding Reference Data and its Importance
Reference data serves as a foundational element within data management, representing a set of permissible values to ensure consistency and accuracy across various systems and processes. It is often used to categorize or classify data, providing a standard vocabulary that facilitates effective communication and interoperability. By maintaining a consistent set of reference data, organizations can ensure that their data is reliable and meaningful, which is crucial for informed decision-making and efficient operations.
- Ensures data consistency across different systems.
- Facilitates interoperability and communication between departments.
- Improves data quality and reliability.
- Supports regulatory compliance by standardizing data formats.
Understanding the importance of reference data is essential for any organization aiming to optimize its data management strategy. It not only helps in reducing errors and discrepancies but also enhances the overall data governance framework. By integrating reference data effectively, companies can streamline operations, improve data analytics, and maintain a competitive edge in the market. Consequently, investing in robust reference data management practices is a strategic move towards achieving operational excellence.
Challenges in Reference Data Integration
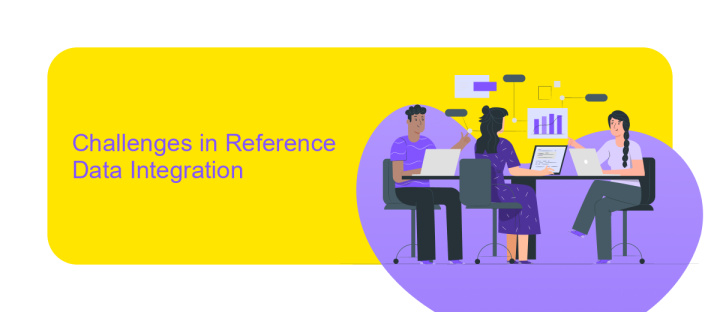
Integrating reference data presents a myriad of challenges, primarily due to the diverse formats and structures in which data is stored across various systems. Ensuring data consistency and accuracy is a significant hurdle, as discrepancies can lead to incorrect analyses and decisions. Moreover, managing the synchronization of data in real-time requires robust systems capable of handling high volumes of data without latency. Another challenge is maintaining data security and privacy, especially when integrating sensitive information from multiple sources, which necessitates stringent compliance with data protection regulations.
Additionally, the complexity of setting up and managing integrations can be daunting. Services like ApiX-Drive offer solutions by simplifying the process of connecting disparate systems, automating data flows, and reducing manual intervention. However, organizations must still address the challenge of ensuring that these integrations are scalable and adaptable to future changes in technology and business needs. Furthermore, aligning different teams and stakeholders to agree on data standards and integration processes remains a critical challenge that requires effective communication and collaboration.
Key Principles and Best Practices for Effective Integration
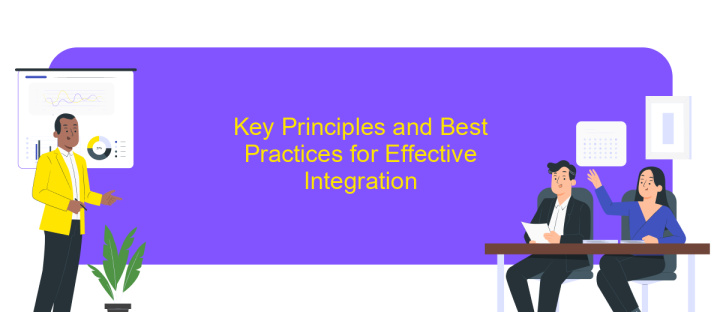
Effective integration of reference data is crucial for ensuring data consistency and accuracy across systems. To achieve this, organizations should focus on several key principles. First, maintain data quality by implementing validation rules and regular audits. Second, ensure data security by applying encryption and access controls. Third, promote data interoperability through the use of standardized formats and protocols.
- Establish a centralized data governance framework to oversee integration processes.
- Utilize automated tools for data mapping and transformation to minimize errors.
- Implement continuous monitoring and feedback loops to address integration issues promptly.
- Foster collaboration between IT and business units to align integration efforts with organizational goals.
- Invest in training programs to enhance staff expertise in data integration technologies.
By adhering to these principles and best practices, organizations can streamline their reference data integration efforts, resulting in improved data quality and operational efficiency. A strategic approach to integration not only supports decision-making processes but also enhances the overall agility and competitiveness of the organization in the data-driven landscape.
Reference Data Integration Architectures and Technologies
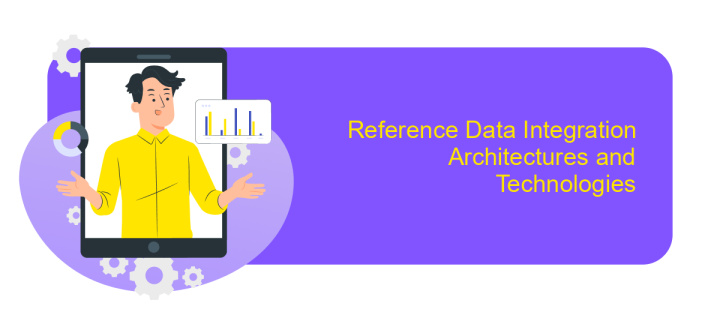
Reference data integration is a critical component of modern data management strategies, enabling organizations to maintain consistency and accuracy across diverse data sources. This integration often involves the alignment of data elements from various systems to create a unified view, supporting decision-making and operational efficiency. Effective architectures and technologies are essential to achieving seamless integration.
Several architectural approaches can be employed for reference data integration, each offering unique advantages. Choosing the right architecture depends on factors such as data volume, complexity, and organizational needs. Technologies that support these architectures play a crucial role in facilitating efficient integration processes.
- Centralized Architecture: Involves a single repository for all reference data, ensuring consistency and ease of management.
- Federated Architecture: Allows for data to remain in its original location while providing a unified view through virtual integration.
- Hybrid Architecture: Combines elements of both centralized and federated approaches, offering flexibility and scalability.
Technologies such as ETL (Extract, Transform, Load) tools, data virtualization platforms, and API-driven integration solutions are commonly used to support these architectures. Selecting the appropriate technology stack is crucial to ensuring robust and scalable reference data integration, ultimately enhancing data governance and operational efficiency.
- Automate the work of an online store or landing
- Empower through integration
- Don't spend money on programmers and integrators
- Save time by automating routine tasks
Measuring Success and Ensuring Data Quality
Measuring the success of reference data integration involves evaluating both the efficiency of the integration process and the quality of the resulting data. Key performance indicators (KPIs) such as data accuracy, consistency, and timeliness are essential metrics. Regular audits and feedback loops should be established to ensure these KPIs are met. Implementing automated tools like ApiX-Drive can streamline the integration process by providing seamless connectivity between diverse data sources, reducing errors, and improving overall data quality.
Ensuring data quality is crucial for maintaining the integrity of integrated systems. This involves establishing robust data governance frameworks that include data cleansing, validation, and enrichment processes. Continuous monitoring and real-time alerts can help identify and rectify data discrepancies promptly. Leveraging platforms like ApiX-Drive can enhance data quality by automating repetitive tasks and ensuring up-to-date information is consistently available, thereby supporting informed decision-making and operational efficiency.
FAQ
What is Reference Data Integration?
Why is Reference Data Integration important?
How can businesses implement Reference Data Integration effectively?
What challenges might organizations face during Reference Data Integration?
How does Reference Data Integration impact data governance?
Routine tasks take a lot of time from employees? Do they burn out, do not have enough working day for the main duties and important things? Do you understand that the only way out of this situation in modern realities is automation? Try Apix-Drive for free and make sure that the online connector in 5 minutes of setting up integration will remove a significant part of the routine from your life and free up time for you and your employees.