Integration of Big Data and the Data Warehouse
The integration of Big Data and the Data Warehouse represents a pivotal advancement in the realm of data management and analytics. As organizations increasingly rely on vast amounts of diverse data to drive decision-making, the seamless fusion of these technologies enables more comprehensive insights, enhanced scalability, and improved efficiency. This article explores the transformative potential of integrating Big Data with traditional data warehousing solutions, highlighting key benefits and challenges.
Introduction: The Convergence of Big Data and Data Warehousing
In today's rapidly evolving digital landscape, the integration of Big Data and data warehousing represents a pivotal shift in how organizations manage and utilize their data resources. As businesses strive to harness the power of data-driven insights, the convergence of these two domains offers unprecedented opportunities for enhanced decision-making and operational efficiency. Big Data, with its vast volume, variety, and velocity, complements the structured and historical data typically stored in data warehouses, creating a comprehensive ecosystem for data analytics.
- Enhanced data processing capabilities
- Improved real-time analytics
- Scalable storage solutions
- Seamless integration of diverse data sources
By merging Big Data technologies with traditional data warehousing, organizations can achieve a more holistic view of their operations and customer interactions. This integration not only supports complex analytical tasks but also drives innovation by enabling businesses to respond swiftly to market changes. As a result, the convergence of Big Data and data warehousing is becoming a cornerstone for enterprises seeking to remain competitive and agile in the digital age.
Understanding the Differences and Synergies
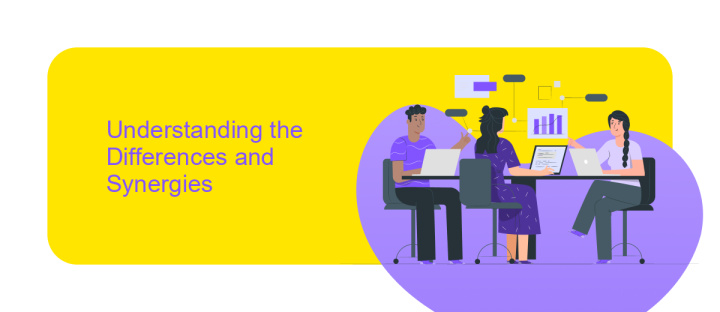
Big Data and Data Warehouses serve distinct yet complementary roles in data management. Big Data is characterized by its vast volume, variety, and velocity, making it ideal for processing and analyzing large datasets in real-time. In contrast, Data Warehouses are structured repositories designed for storing historical data and supporting complex queries and reporting. Understanding these differences is crucial for organizations aiming to leverage both technologies effectively. While Big Data excels in handling unstructured data and real-time analytics, Data Warehouses provide a stable environment for structured data and long-term analysis.
The synergy between Big Data and Data Warehouses can be harnessed to enhance decision-making processes. By integrating these systems, businesses can achieve a comprehensive view of their data landscape. Tools like ApiX-Drive facilitate seamless integration, allowing for automated data flow between Big Data platforms and Data Warehouses. This integration enables organizations to combine real-time insights with historical data analysis, driving more informed business strategies. Leveraging these synergies ensures that companies can maximize the value of their data, leading to improved performance and competitive advantage.
Architectures and Approaches for Integration
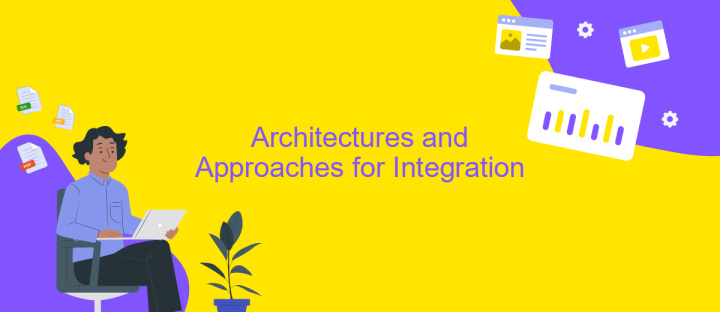
Integrating Big Data with traditional data warehouses requires a strategic approach to handle diverse data types, volumes, and velocities. The architecture must support scalability, flexibility, and real-time processing to ensure seamless integration. Organizations often face challenges in merging structured and unstructured data, necessitating innovative solutions to maintain data quality and accessibility.
- Hybrid Data Architecture: Combines the strengths of data warehouses and Big Data platforms, allowing for both batch and real-time processing.
- Data Lakes: Serve as centralized repositories for raw data, providing a foundation for advanced analytics and machine learning.
- ETL and ELT Processes: Modernize traditional Extract, Transform, Load (ETL) processes to include Extract, Load, Transform (ELT) for greater efficiency in handling large datasets.
Successful integration of Big Data and data warehouses hinges on selecting the right architecture and approach tailored to organizational needs. By leveraging hybrid models, data lakes, and advanced ETL/ELT processes, businesses can achieve a unified data ecosystem. This enhances decision-making capabilities and drives innovation, ensuring that data remains a valuable asset in the competitive landscape.
Benefits and Challenges of Big Data and Data Warehouse Integration
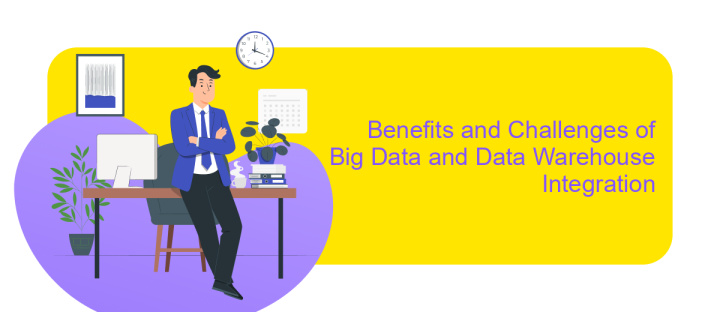
Integrating Big Data with traditional data warehouses offers a potent combination that can drive significant business value. By merging the vast, unstructured datasets of Big Data with the structured, reliable data of warehouses, organizations can gain comprehensive insights and make informed decisions. This integration allows businesses to leverage advanced analytics and predictive modeling, enhancing their ability to respond to market trends and customer needs swiftly.
However, this integration is not without its challenges. The complexity of managing and processing large volumes of data can strain existing IT infrastructures. Furthermore, ensuring data quality and consistency between disparate systems can be a daunting task. Organizations must also address security and privacy concerns, as integrating diverse data sources can increase vulnerability to breaches.
- Enhanced decision-making capabilities
- Improved data accessibility and sharing
- Challenges in data governance and quality
- Increased infrastructure and maintenance costs
Despite these challenges, the integration of Big Data and data warehouses is a strategic move for organizations aiming to stay competitive. By carefully planning and addressing potential issues, businesses can harness the full potential of their data, driving innovation and efficiency across operations.
Future Trends and Considerations
As the landscape of data management continues to evolve, the integration of Big Data with traditional data warehouses is expected to become even more seamless and sophisticated. Emerging technologies such as artificial intelligence and machine learning will play pivotal roles in automating data integration processes, enhancing data accuracy, and providing real-time analytics. Organizations will increasingly rely on cloud-based solutions to manage their data ecosystems, offering scalability and flexibility. The shift towards hybrid models that combine on-premise and cloud solutions will also gain momentum, catering to diverse business needs and regulatory requirements.
In this dynamic environment, tools like ApiX-Drive will become indispensable for businesses seeking efficient data integration. ApiX-Drive offers automated solutions that simplify the integration of various data sources, reducing the need for extensive coding and manual intervention. As data volumes continue to grow exponentially, the ability to seamlessly connect and manage diverse datasets will be crucial. Future trends will likely focus on enhancing data security, privacy, and compliance, ensuring that businesses not only harness the power of Big Data but do so responsibly and ethically.
FAQ
What is the role of Big Data in a Data Warehouse?
How can businesses integrate Big Data with their existing Data Warehouse?
What are the challenges in integrating Big Data with a Data Warehouse?
How can automated data integration tools assist in integrating Big Data with a Data Warehouse?
What are the benefits of integrating Big Data with a Data Warehouse?
Do you want to achieve your goals in business, career and life faster and better? Do it with ApiX-Drive – a tool that will remove a significant part of the routine from workflows and free up additional time to achieve your goals. Test the capabilities of Apix-Drive for free – see for yourself the effectiveness of the tool.