ETL vs Data Lake
In the evolving landscape of data management, ETL (Extract, Transform, Load) and Data Lakes represent two distinct approaches to handling large volumes of information. ETL processes structure and cleanse data for specific uses, while Data Lakes offer a more flexible, raw data repository. Understanding the differences between these methods is crucial for optimizing data strategy and achieving business goals.
Introduction to ETL and Data Lakes
ETL (Extract, Transform, Load) and Data Lakes are two fundamental concepts in modern data management. ETL is a process that involves extracting data from various sources, transforming it into a suitable format, and loading it into a target database or data warehouse. Data Lakes, on the other hand, are centralized repositories that allow you to store all your structured and unstructured data at any scale.
- ETL Process: Extract, Transform, Load
- Data Lake: Centralized repository for all data types
- ApiX-Drive: Service for automating data integration
While ETL is essential for structured data processing and analytics, Data Lakes offer flexibility for storing a wide variety of data types. Services like ApiX-Drive facilitate seamless integration between different data sources and destinations, making it easier to automate ETL processes and manage data within Data Lakes. Understanding the strengths and use cases of both ETL and Data Lakes can help organizations make informed decisions about their data management strategies.
Comparison of ETL and Data Lakes
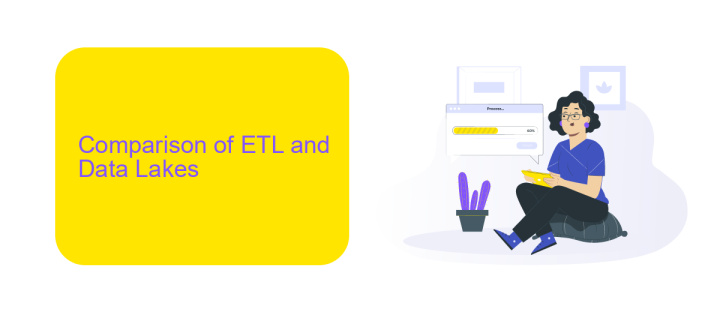
ETL (Extract, Transform, Load) and Data Lakes serve different purposes in data management but often complement each other. ETL is a process-oriented approach where data is extracted from various sources, transformed into a suitable format, and then loaded into a target database or data warehouse. This method ensures that the data is clean, consistent, and ready for analysis. ETL is particularly useful for structured data and is often employed in traditional business intelligence and reporting systems.
On the other hand, Data Lakes are storage repositories that hold vast amounts of raw data in its native format until it is needed. They are highly scalable and can store structured, semi-structured, and unstructured data, making them ideal for big data and advanced analytics applications. While ETL processes can be used to load data into Data Lakes, tools like ApiX-Drive can simplify the integration and automation of data flows between various systems and the Data Lake. This flexibility allows organizations to leverage both ETL and Data Lakes to meet diverse data management and analytics needs.
Advantages and Disadvantages of ETL and Data Lakes
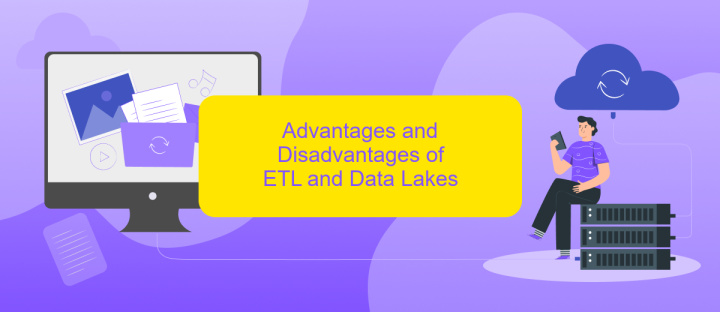
ETL (Extract, Transform, Load) and Data Lakes are two distinct approaches to data management, each with its own set of advantages and disadvantages.
- ETL Advantages:
- Data Quality: ETL processes ensure data is clean and well-structured before loading.
- Integration: Easily integrates with existing systems and databases.
- Performance: Optimized for faster query performance.
- ETL Disadvantages:
- Complexity: Requires significant setup and maintenance.
- Cost: Can be expensive in terms of both time and resources.
- Flexibility: Less adaptable to changes in data sources and structures.
- Data Lake Advantages:
- Scalability: Can store vast amounts of data in various formats.
- Flexibility: Easily accommodates changes in data sources and structures.
- Cost-Effective: Generally cheaper for storing large volumes of data.
- Data Lake Disadvantages:
- Data Quality: Lack of structure can lead to data quality issues.
- Complexity: Requires sophisticated tools and skills to manage.
- Performance: Slower query performance compared to ETL.
Choosing between ETL and Data Lakes depends on the specific needs of your organization. For example, ApiX-Drive can help streamline ETL processes, making it easier to integrate various data sources. On the other hand, Data Lakes offer greater flexibility and scalability, which can be advantageous for handling diverse and large datasets.
Use Cases for ETL and Data Lakes
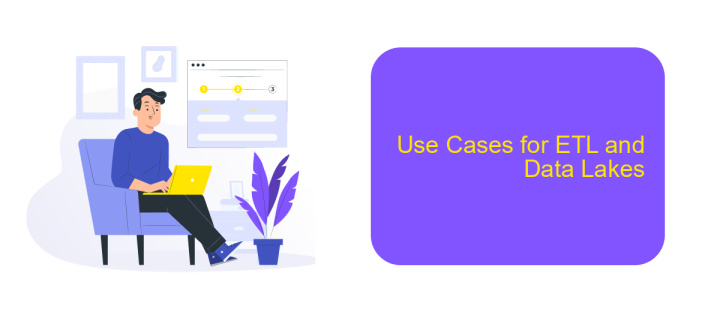
ETL (Extract, Transform, Load) and Data Lakes are essential components in modern data management strategies. ETL processes are primarily used to integrate data from various sources, transform it into a usable format, and load it into data warehouses for analysis. Data Lakes, on the other hand, are designed to store vast amounts of raw, unstructured data for future processing and analysis.
Use cases for ETL typically involve scenarios where structured data needs to be consolidated and transformed for business intelligence and reporting purposes. ETL is particularly useful in industries that rely heavily on data accuracy and consistency, such as finance, healthcare, and retail.
- Data consolidation from multiple sources
- Data transformation for business analytics
- Ensuring data quality and consistency
- Loading data into data warehouses
Data Lakes are ideal for organizations that deal with large volumes of unstructured data, such as social media feeds, sensor data, and log files. They allow for flexible data storage and are useful for advanced analytics, machine learning, and big data applications. Services like ApiX-Drive can be used to streamline the integration of various data sources into both ETL pipelines and Data Lakes, enhancing data accessibility and usability.
- Automate the work of an online store or landing
- Empower through integration
- Don't spend money on programmers and integrators
- Save time by automating routine tasks
Future of ETL and Data Lakes
The future of ETL (Extract, Transform, Load) and Data Lakes is poised for significant evolution as organizations increasingly rely on data-driven decision-making. ETL processes are becoming more automated and intelligent, leveraging machine learning algorithms to enhance data quality and transformation accuracy. This shift is reducing the manual effort required and increasing the efficiency of data integration. On the other hand, Data Lakes are evolving to handle more complex and diverse data types, supporting real-time analytics and advanced data processing capabilities. The integration of ETL and Data Lakes is crucial for creating a seamless data ecosystem that can support various analytical needs.
In this evolving landscape, services like ApiX-Drive play a pivotal role by simplifying the integration of various data sources and automating data workflows. ApiX-Drive allows businesses to connect multiple applications and services without extensive coding, facilitating smoother ETL processes. This not only accelerates data integration but also ensures that data is consistently up-to-date and accessible for analysis. As the demand for real-time data processing and analytics grows, tools like ApiX-Drive will become indispensable, enabling organizations to stay agile and competitive in the data-centric future.
FAQ
What is the main difference between ETL and a Data Lake?
Can ETL processes be automated?
Is a Data Lake a replacement for a Data Warehouse?
How do you ensure data quality in a Data Lake?
What are the benefits of using a Data Lake?
Apix-Drive will help optimize business processes, save you from a lot of routine tasks and unnecessary costs for automation, attracting additional specialists. Try setting up a free test connection with ApiX-Drive and see for yourself. Now you have to think about where to invest the freed time and money!